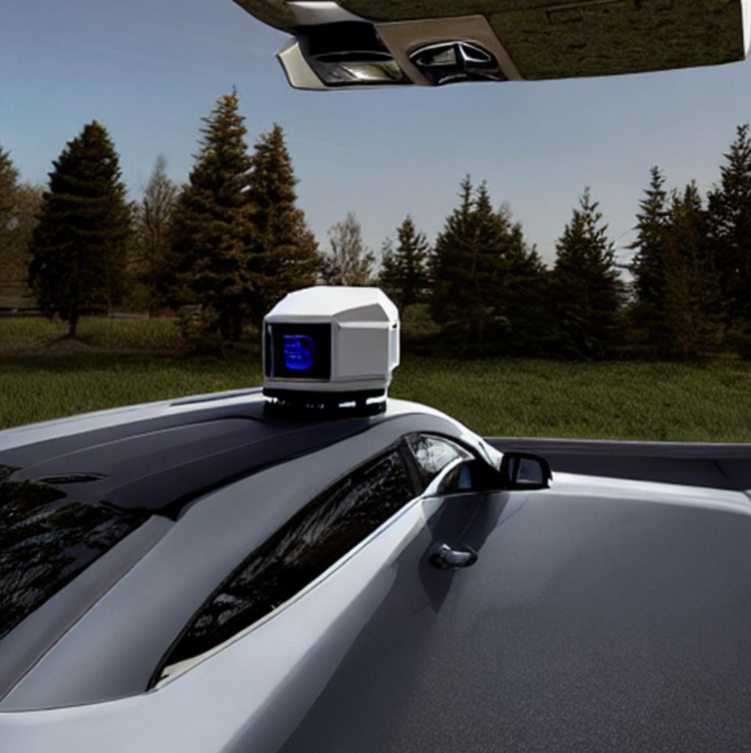
Lidar sensors tend to be relatively expensive compared to other sensing technologies. The intricate design, the high-quality components, and the precision required for accurate measurements contribute to a higher price. Still, more and more automotive companies consider adopting Lidar sensors for their ADAS and Autonomous Driving platforms. A Lidar sensor can be bulky and heavy, especially the mechanical spinning or scanning Lidar types that require moving parts. This can be a limitation for applications where size and weight constraints are critical such as autonomous vehicles. Additonally, any mechanical systems is vulnerable to pyhsical srtress, like strong vibrations casued e.g., by bad road conditions. Although lidar sensors can provide highly accurate measurements, their effective range is often limited compared to other sensing technologies. For example, long-range Lidar sensors are available but tend to be significantly more expensive. In situations where long-distance sensing is required, such as highway monitoring or aerial mapping, this limited range can be a drawback. The vulnerability to weather conditions is a big drawback of Lidar systems. Adverse weather conditions such as rain, fog, or snow can interfere with lidar sensor performance. The laser beams can scatter or get absorbed by the particles in the air, leading to reduced accuracy or even complete failure in extreme cases. This vulnerability to weather limits the reliability of lidar in certain environments. Then. Lidar sensors can be sensitive to external light sources, particularly when operating in the visible spectrum. Bright sunlight or other intense light sources can interfere with the sensor readings and affect its performance and accuracy. Today’s Lidar sensors generate huge amounts of point cloud data that require significant computational power and processing time to convert into actionable information. Real-time processing of lidar data can be demanding, especially for applications that require quick decision-making or response times. Finally, the Lidar industry lacks standardization in terms of data formats and processing algorithms. Different lidar systems may generate data in various formats, making it challenging to integrate data from different sources or utilize off-the-shelf software tools. This lack of standardization impedes interoperability and increase engineering and development costs.